Abstract
Understanding how gene expression in single cells progresses over time is vital for revealing the mechanisms governing cell fate transitions. RNA velocity, which infers immediate changes in gene expression by comparing levels of new (unspliced) versus mature (spliced) transcripts, represents an important advance to these efforts. A key question remaining is whether it is possible to predict the most probable cell state backward or forward over arbitrary time scales.
In this webinar, Xiaojie Qiu of the University of California, San Francisco, shares an inclusive model, termed Dynamo, capable of predicting cell states over extended time periods. The model incorporates promoter state switching, transcription, splicing, translation, and RNA/protein degradation by taking advantage of single-cell RNA-seq data and transcriptome/proteome co-assay measurements.
Dr. Qiu demonstrate how the Dyamo model can be used to infer the entire kinetic behavior of a cell and will show that it is possible to analytically reconstruct the transcriptomic vector field from sparse and noisy vector samples generated by single-cell experiments, especially those produced from metabolic labeling based scRNA-seq (i.e scSLAM-seq, NASC-seq, sci-fate or scNT-seq).
In this webinar you will learn:
- How to use Dynamo to perform RNA velocity analysis and vector field reconstruction
- Details of an inclusive model capable of predicting cell states over extended time periods
- How to analytically reconstruct the transcriptomic vector field from sparse and noisy vector samples generated by single-cell experiments
Speaker’s biography
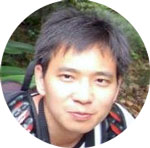
Dr. Xiaojie Qiu is a postdoctoral research fellow in Jonathan Weissman’s lab at UCSF, focusing on developing new single-cell genomics techniques (i.e. metabolic labeling based single-cell assays) and building novel computational frameworks to understand the fate of cells, and to predict and manipulate it. Xiaojie did his Ph.D. at the University of Washington under the supervision of Dr. Cole Trapnell. Xiaojie’s Ph.D. work made substantial contributions to the field of single-cell genomics, exemplified by the development of Monocle 2/3, which can accurately and robustly reconstruct complex developmental trajectories from scRNA-seq data. In close collaboration with Dr. Sreeram Kannan, he also developed Scribe to detect casual interactions from single-cell time-series datasets.